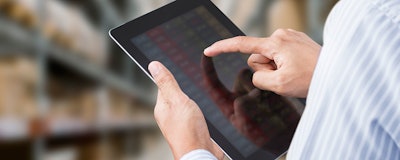
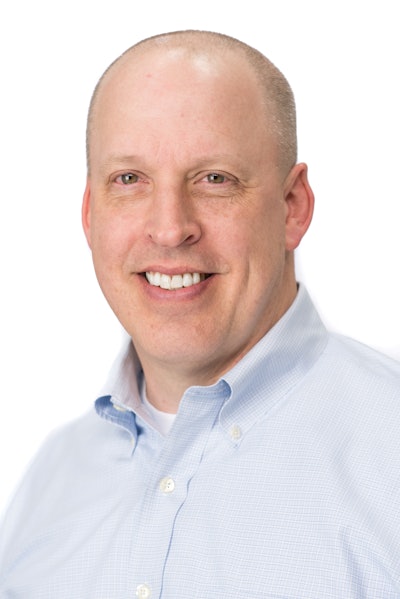
Just a few decades ago, manufacturers were starved for information about their production processes and overall quality. Fast-forward to today and it is quite the opposite. Thanks to the advent of automation, handheld devices, and the Internet of Things (IoT), fully-automated data collection is now possible. It not only makes getting quality data easy, but also eliminates the chances of human error and frees up shop-floor operators to focus on more critical aspects of operations than data entry.
However, the outcome of all this automation and ease is organizations now have too much data on their hands. Because of automation, many want to gather as much data as they can. They want process data like temperatures, feeds and speeds, and product quality data like dimensional measurements and weights—every millisecond, around the clock. Manufacturers are, in effect, drowning in data.
The truth is, collecting data for the sake of having it does not lead to improved processes or product quality. The insight gleaned from the data is what actually benefits the business. And manufacturers do not need every measurement, every millisecond to get it. What they need to know is what data is important, how much to collect, and how to extract meaningful intelligence from it.
Should We Collect the Data?
While the ability to gather comprehensive process data is exciting, manufacturers should first ask themselves the following questions before risking “data gluttony.”
- Why do we need to gather this data?
- Is it to solve a short-term problem?
- Is it to fulfill a long-term strategic goal?
- How will we use the data after collection?
- Who will evaluate the data? And how?
- What is a reasonable amount of data to collect?
- How frequently do we need to collect the data?
By asking questions like these, manufacturers can form rational data collection plans with reasonable frequencies of data sampling, rather than just collect mountains of numbers that no one has time to review. Storing excessive amounts of data can quickly get expensive. If a plant collects every millisecond of data for just one feature, then imagine that extrapolated across an entire plant—then the whole enterprise. It would fill up lots of hard drives in no time. Instead, there should be a focus on how much data is needed, not how much is wanted.
What Will We Do With the Data?
Collected data must serve a purpose. After all, what manufacturers truly need is not data, but information. They want information that will tell a story that can drive action and lead to operational improvements and bottom-line results. And it can come from both large and small datasets. Sometimes the most innocuous datasets can reveal extraordinary stories that generate millions of dollars in savings.
The key is to convert data into intelligence. Not by leaving it sitting in a database. Instead, manufacturing intelligence is extracted from data by aggregating, summarizing, and analyzing it through statistical techniques, then repeating the process on a regular basis. This is the formula that so many companies have used to generate millions in savings. To be successful in this endeavor, manufacturers must regularly set aside time to do the analyses. Such scheduled, recurring, “information extraction” meetings are critical for driving continuous improvement and operational excellence through the use of data.
In these meetings, managers, engineers, and quality professionals step back from their daily tasks to band together and dig into the data gathered on the shop floor. They compare performance across manufacturing lines, product codes, and even plants. Their goal is to better understand what the data is telling them and uncover information that they never knew was there.
Some companies apply the formula monthly, others weekly or quarterly. But their success comes from the following commitments:
- To collect data for very specific reasons
- To analyze the collected data
- To act upon the information found in the data
- To repeat the process in order to:
- Continually improve upon processes
- Reduce costs and improve product quality
Where Do We Go From Here?
Moving forward, the first priority is to collect only the data that is needed—not everything. Second, consider consolidating all of the data into a centralized data repository. Only then can manufacturers perform the comparisons and analyses across production lines, plants, regions; between product codes, vendors, shifts, and more.
With all the data in one place—including multiple plants and suppliers—manufacturers can aggregate, summarize, and roll-up the data at any level, including within each plant and at the regional level. They can even provide reporting for the executive or C-suite level. With data residing in one single repository, it is possible to mine meaningful insights across all operations, across the entire enterprise.
Organizations can then prioritize improvement opportunities based on which ones will generate positive impacts in the shortest amount of time. Only the right tools can support such analyses and convert the aggregated data to actionable intelligence that can positively transform process performance, product quality, and the bottom line.
Douglas Fair is COO of InfinityQS International, Inc.