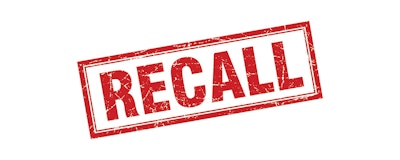
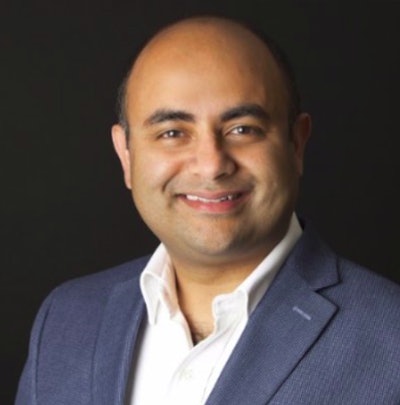
Just days into 2018, yet another major company was forced to issue a significant product recall. This time it was HP, which recalled 50,000 computer batteries that were overheating and causing burn hazards for customers.
Disasters like this one have become increasingly commonplace in today’s business world. To keep up with rising demand, enterprises are being challenged to work faster while also maintaining high efficiency and cost-effectiveness. However, even the smallest mishap in the manufacturing process can lead to faulty products that necessitate costly product recalls.
The Challenges of Modern Manufacturing
While product recalls are by no means new, they are more pressing than ever. With the manufacturing process becoming more complex, recall costs have skyrocketed and it’s even harder to recover from a recall as well.
Many businesses thought the evolution of the Industrial Internet of Things (IIoT) over the past decade would help shed light on their manufacturing activities. With greater insight into their machine performance, fluctuations and changes that were once undetectable can now be observed and recorded on a real-time basis. However, despite enterprises looking at IIoT as a game-changer, many still struggle to get the optimal performance out of their machines.
This is for two reasons. First, legacy machines can hinder businesses from capitalizing on the IIoT boom. While intelligent sensors can be added to some of these assets to bridge the gap, the data output may still not be sufficient for accurately predicting anomalies.
The second problem lies in how manufacturers use the information they are collecting. The IIoT gives businesses all sorts of unique information about how their machines function. This is great in theory, but applying old and traditional approaches of data analysis in this new world of IIoT won’t enable businesses to tap into the true potential. Many teams take a machine learning approach called supervised learning, which builds models trained on historical failure or fault data. Other teams simply write rules to generate alerts and notifications when past failures or faults are primed to reoccur. However, these approaches only accentuate what many businesses are already good at — preventative maintenance.
The problem is that most issues that cause recalls or major catastrophes are not reoccurring events — they are new events, caused by unknown or never-before-seen causes that traditional models or domain expertise can’t detect. These “unknown unknowns” are the business threats that require a scalable solution.
The Cognitive Advantage
Because many businesses still take a supervised approach to anomaly detection and prediction, they are generally limited in terms of what they can do. They are forced to be reactive by nature, implementing rules designed to stop known anomalies from reoccurring.
This is where cognitive anomaly detection and prediction comes into play, combining the best of unsupervised and supervised machine learning approaches. Through unsupervised machine learning, the cognitive approach enables the business to learn in real time the “normal behavior” of the asset in a given environmental and operating condition, which can help identify deviations. Supervised learning can then be used to look for any similarities in deviation patterns from past problems and automatically characterize which of those deviations could be early signals of a potential problem — regardless of whether it’s known or unknown.
With the evolution of sensor information and IIoT, what was once impossible has now become possible. Companies can now track every component of a heavy machine and identify highly localized signals that pinpoint potential causes for concern.
With a cognitive approach, enterprises can use a machine-first technique to automatically find data patterns or groupings at scale. These patterns would otherwise be hidden in the massive volumes of generated sensor data and would have been humanly impossible to unearth in time to prevent major issues. By leveraging cognitive anomaly detection and prediction, businesses are better equipped to analyze the data coming from all their assets and identify the unknown variables that are responsible for many of the defects that lead to recalls or failure events.
4 Steps for Preventing Recalls with Cognitive Anomaly Detection and Prediction
If businesses want to get serious about their recall prevention strategies, it starts by realizing the importance of the unknown unknowns in the manufacturing process. Here are the four steps businesses need to take to embrace the cognitive approach to anomaly detection and prediction and identify these unknowns:
- Make sure your assets and equipment are properly instrumented. Every machine needs to have the right sensors attached to it so businesses can get the data they need to identify performance anomalies.
- Perfect your data management processes. All the data produced by sensors is critical for observing patterns and creating predictive maintenance models that will prevent recalls. Enterprises need to ensure they are collecting, processing, cleansing, storing and using their data effectively at scale — otherwise, all the analysis and model building done using partial or dirty data will provide limited or no value.
- Learn how to leverage unsupervised learning techniques. Enterprises need to be able to identify normal operating conditions and then separate out deviations. This must be done on a per-asset level. Generic models covering all assets simply aren’t specific enough to capture the individual characteristics of an asset given its unique environmental and operating conditions.
- Create a process for acting on predictions and detections in a timely fashion. Having a simple dashboard application often isn’t enough — events can easily go missed. Ideally, businesses should be able to automate a response to their predictions and detections. For example, a service ticket is raised automatically on detection or a self-maintenance routine is triggered wherever possible.
Despite the rising costs associated with recalls, many businesses still do not have an adequate approach to recall prevention. The evolution of IIoT and sensor technology in combination with cognitive machine learning and artificial intelligence in recent years has empowered manufactures. But if they want to fully capitalize on their data and maximize their manufacturing performance, they need to take a proactive cognitive approach.
Ruban Phukan is Co-founder of DataRPM & VP Product at Progress.