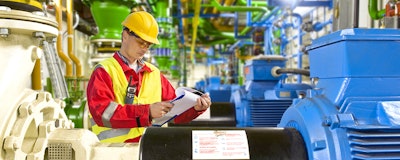
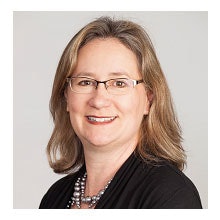
How do you think doctors know that high blood pressure can lead to critical health issues and conditions? And how did the medical field come up with the numbers that distinguish high blood pressure from normal blood pressure readings?
The answer: medical science kept records over time on these readings and the outcomes from them, and performed analytics on the data that revealed the association of high blood pressure to deadly outcomes. The medical field thus became able to predict and prevent or treat certain related health conditions based on a data point. The same is possible with manufacturing assets.
Most manufacturing companies have already moved toward a preventive maintenance approach, with scheduled maintenance at set intervals, usually determined by time. These schedules often begin with tribal knowledge, gleaned from years of hands-on experience. But excessive maintenance can be a drain on resources, since 30 percent of preventive maintenance activities are carried out too frequently.
Moving to more advanced maintenance strategies may seem like a huge leap for small to mid-size manufacturers. However, to reduce the intimidation factor, consider maintenance as a continuum of gradual transitions based on information from the machines.
Listening to the Machines
Research by IBM found that 89 percent of asset failures occur at random, and those are difficult to prevent with planned maintenance. Condition-based maintenance can provide an appropriate strategy for these random failures. Asset conditions trigger maintenance with enough lead time before failure, so work can be completed before performance falls below tolerance.
To begin moving toward this usage-driven model of preventive maintenance, it is critical to have data. Identify the appropriate operational metric for your production. It is important that this data be obtained from the assets. Ideally sensors would be integrated with the EAM, although operator input is sufficient. Production is not interrupted because data is gathered while the equipment is running. Deploying sensors on the line is not as difficult as you might imagine. There are many wireless sensors available, at reasonable costs that give you the freedom to move them from asset to asset if necessary, and to deploy without disrupting your operations to wire a network to capture the data. Sensors are available to capture production counts, rejects, temperatures, humidity and pressures.
Applying What We’ve Learned
As more and more information is available to analyze, a reliability engineer can begin to identify trends and patterns. Each data point collected tends to lead to additional questions, and to gathering more information to create a more complete picture of each asset’s specific maintenance needs.
The benefit of this approach is that maintenance is based on empirical evidence rather than tribal knowledge. Going beyond the surface to uncover the root cause of issues will help determine the source of chronic or expensive breakdowns. With that knowledge, a course of action can be set to prevent recurrences.
Turning Over the Wheel
The final, most advanced form of maintenance removes the human element almost entirely. Instead, it relies on a combination of maintenance solutions, planning and production systems, critical equipment sensors and advanced analytics to create the smart, connected factory. The constant flow of data from connected assets and related systems means changing conditions can be addressed in real time, with systems automatically generating maintenance tickets, ordering necessary parts and adjusting production schedules if they’re impacted.
While this stage of proactive maintenance may seem unattainable for small and mid-size manufacturing companies, it is important to continue to aspire for more advanced strategies. Changes do not have to be made all at once throughout an entire facility. Incremental improvement in one area can provide the impetus to gradually expand the scope of the initiative. If you do not start to get comfortable now with using data from your assets and what it may be telling you, then you will not be able to advance your organization as these technologies become more widely adopted.
There is no one maintenance strategy for all situations and all companies. The goal of preventive and proactive maintenance is to have the right work done on the right schedule. The difference between the two is knowing exactly how and when to act. Rather than relying on tribal knowledge and rigid schedules, empirical data derived from the assets themselves can determine what action needs to be taken at any given time. The machines are talking and passing along important information to us; we need to become better equipped at listening and acting on that information.
Kay Jenkins is Director of Aptean TabWare EAM.