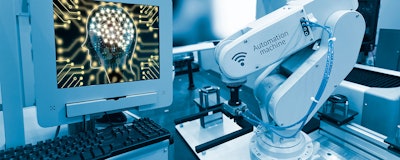
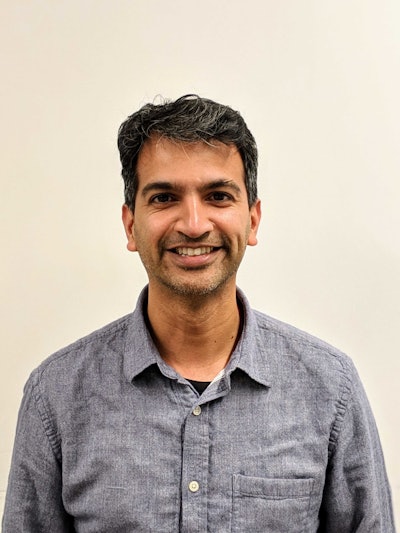
A common pitfall a lot of machine learning (ML) companies run into is mistaking data as knowledge. Several enterprises think that having a lot of data makes them ripe for harvesting insights instantly through AI and ML techniques. It is not entirely true.
Data is not equal to knowledge, or more precisely, not the knowledge you think it equals.
Ernesto Miguel, 47 is a plant operator in a leading cement company. He has spent the last three decades working in the same cement plant. He knows each and every machine in his cement plant intimately. From the sound they make, he can tell what can be wrong. He is a champion in ensuring that the machines operate at their highest efficiency.
Ernesto has tricks up his sleeve that he has developed over the last thirty years. For instance, he can listen to the hum of a cement cooler and get a sense of its health and in many cases can adjust the grate pressure to maximize the operating efficiency. Similarly, for other systems, he is proactive in registering the operational signs and controlling various parameters in the plant to ensure the health of the equipment.
Ernesto is not alone in his domain expertise. A lot of industrial workers are.
Recently Ernesto's company has brought on an AI startup to improve the plant operations and optimize revenue and reduce plant floor costs. With the Industrial Internet of Things (IIoT) adoption a few years ago, the plant has year’s worth of data capturing the operation of each and every equipment in the cement plant. This was a problem ripe for AI. Or that is what everyone thought.
The AI engineers working on this problem built a model of a plant equipment (cooler) looking at the time series data of how the equipment behaved over the last two years. They identified the correlation between different sensors and equipment and baked it in the Neural Network model. The model seemed to perfectly capture the operation of the cooler equipment. The data seemed to mirror their understanding of the cooler's operation. Data, they thought, captured the equipment operation accurately and this was the first step in the experiment of letting the AI system control the plant directly without input from the plant operator.
Think about the implications of this. In a scenario where people are able to accurately understand and model the real world, they can liberate the experienced operators like Ernesto from routine manual work and boost productivity by giving them AI tools that they can confidently rely on.
It was a big moment when the first AI solution was deployed in the cement plant. There was a safety switch included for the plant operator to intervene if something went wrong. We don't yet live in a world where we can trust machines completely. For good reason. The first exercise was to run the solution overnight where the AI system monitored the cooler and was responsible for keeping it within safe bounds. To the delight of everyone, the system successfully ran overnight. But that joy was short-lived when the first weaknesses in the model started appearing.
The cooler temperature was increasing. And the model with an established correlation between the temperature and fan speed, kept increasing the fan speed. In the meantime, the back-grate pressure increased above the safe value. But the model had identified no correlation between the back-grate pressure and the temperature and felt no need to adjust the back-grate pressure in its objective of bringing down the cooler temperature. The plant operator overrode the control and shut off the AI model.
An experienced plant control would have immediately responded to the increasing back-grate pressure as it is detrimental to the cooler's operation. How did the AI model miss this?
In his thirty years, Ernesto never had to wait for the grate pressure to build up before reacting. He just knew when the pressure would build up and pro-actively controlled the parameters to ensure that the grate pressure would never cross a safe bound. By merely looking at the data, there was no way for the ML engineers to determine this. The data alone without context would tell them that the grate pressure would never be a problem.
This is the fundamental problem with some of the ML approaches in the world today. Engineers falsely think that they can model the real world by looking at the data. When skilled decision making is involved, this assumption is no longer valid and the model they are able to create is a weak model of what the real world actually is.
Data does not translate directly to knowledge. Data contains patterns and signatures that might not be obvious directly. Using that data blindly to solve real-world problems will lead humanity into dangerous territories. Sensemaking of the data and identifying the right correlations among the different data dimensions is a problem that engineers are yet to solve elegantly as a community.
How do we tackle this? I am confident that there are a lot of opportunities to redefine how people aggregate data in the first place where context is baked in. But a big challenge in applied ML is going to have engineers work with the existing data, which might be incorrect or incomplete. This will create the risk of ML models not adequately equipped to solve the problems they are intended to solve. There is no other option except to work with domain experts and spend extra effort in understanding the data without making assumptions about its validity and completeness in capturing the real world.
Another thing that works to solve this problem is to co-design ML solutions with expert humans in loop. The ML solutions deployed should aspire to augment the capabilities of humans and will not be able to autonomously act, at least, not yet. This calls for embracing co-design for all applied AI/ML products, a well understood design thinking approach where the product designed is not built in a vacuum but with extreme customer engagement.
Eventually, data acquisition techniques will allow engineers to model the real world accurately. Until then, engineers need to ensure that there are enough safety checks built into AI solutions to respond, learn, and iterate.
Saranyan Vigraham is vice president of engineering at Petuum.