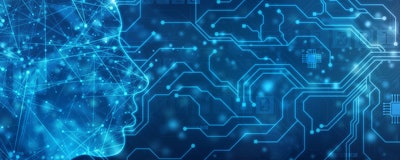
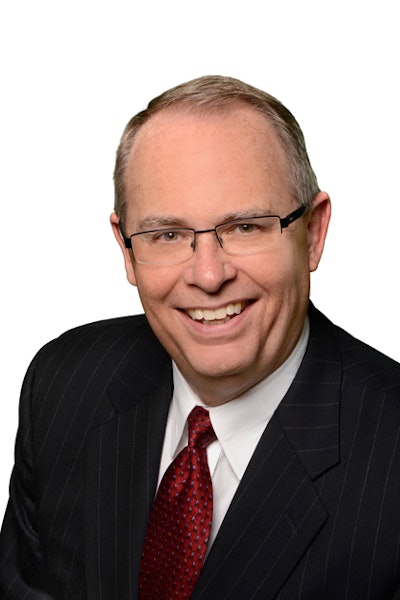
There are plenty of areas where artificial intelligence is helpful and extremely useful. Areas like web marketing, retail product recommendations and consumer help desks can all streamline processes in addition to other benefits when using AI.
However, while these areas reap the benefits, is AI the right choice in industrial applications like the Industrial Internet of Things (IIoT) or is there a better option? Many AI methods are self-taught, so they avoid the need for process mapping and other tedious analytical processes, making it seem to be the right fit for IIoT. Yet, only a few methods will apply. The most useful methods are not greedy for impossible amounts of data. They focus machine learning in explainable ways. The rest will fail badly and there are three reasons why.
No. 1 - Complex Failure
Twenty-first century production is complex. Each process has thousands, or even millions, of steps. Each of which has variability depending on equipment, people and the item being processed.
Most AI cannot comprehend big processes like this, but even for simple systems, AI fails. For example, the least complex machine is a simple pump attached to an electric motor. There are 50 ways this system can fail with more than 50 causes of failure. This means there are 2,500 different possibilities for failure on this single asset. How long will data need to be collected for the AI to gain enough insight to diagnose the issue or correct the impending failure? It could take years, and even then the AI may not be able to gather all the data.
If all the data could be collected to train the AI, the processing time to solve the problem would be extensive. To get around this, Big Data AI methods use a trick called dimensionality reduction. This trick allows the AI to find correlations and lumps them together to create one variable, simplifying the process. But what happens when a problem occurs and the items are not correlated? The AI will not be able to detect what is actually wrong or provide a prescription to fix the problem.
Can AI support complex failure analysis? Yes, but the most popular brute force methods optimized for consumer applications are not the answer. Hybrid AI, blending machine learning, expert systems and other techniques is a better answer in most cases.
No. 2 - Ongoing Operations Evolution
The configuration of capital assets, the workforce and the nature of production are just three operation processes which are constantly being changed by production managers. Since Big Data AI bases its calculations over long-term observations and statistical correlations, it has trouble telling the difference between good and bad changes.
This adds another level of complexity Big Data AI cannot fully grasp.
Here too, a hybrid approach is more robust.
No. 3 - Poor Time-to-Value
Even in situations where traditional AI can work in manufacturing, it can take a long time for it to collect data and then learn what to do with the data. Waiting months to even more than a year is not practical when solutions are needed right now. Additionally, the company will be paying for this service before the AI is able to prescribe a solution, further decreasing the return on investment.
A hybrid AI approach using predictive and prescriptive analytics solutions are more effective for IIoT than traditional AI. The latter has its place, but for much of IIoT, it is too slow and too expensive. Additionally, traditional AI can be too cryptic when providing a solution. When AI algorithms complete training, their original creators often cannot explain how the AI produces its answers. Since human decision makers cannot understand the process, they are often reluctant to accept the AI’s conclusions.
On the other hand, the best analytics companies break big questions down into smaller, easier-to-answer questions. This creates solutions which are easy to understand. Interpreting these predictions are simple and straight forward, so humans are more likely to act and act faster.
For example, one of the biggest opportunities for using analytics in the manufacturing industry is in lean production improvement. Instead of waiting for machines to fail and then having to pay for costly repairs, operators can use analytics to forecast needed repairs and schedule crews before failures shut down production. This predictive approach lowers equipment operating costs, improves safety, increases production and decreases downtime.
Lone Star worked with an after-market automotive parts manufacturer who was experiencing difficulty with its two-part reaction injection molding (RIM) mixing nozzles clogging. When this happened, the manufacturer faced excessive costs in increased downtime, scrap parts and labor touch hours.
The analytics team took the limited amount of sensor data available and worked with subject matter experts to better understand the factory and the machine. This enabled a real-time predictive model to forecast nozzle clogging and alert operators when it was likely to occur. Because of this, instead of waiting for the machine to shut down, the operator was able to address the problem before it occurred.
This solution reduced nozzle changes, eliminated nozzle clogs and improved the quality of products by reducing the scrap that previously occurred. Overall, it saved the manufacturer around $450,000 annually and increased the machine’s performance by 7 percent. The company also saw a ROI in less than three months. If Big Data AI was set to solve this problem, it would still be sourcing data, whereas Lone Star was able to go from contract to completion within five weeks, including integration of the solution.
The solution did include some AI features, but it was not a brute force, big data design.
Instead of looking to consumer and advertising AI as a solution for all problems, decision makers need to see how it can be used effectively or if it can be replaced with a better approach.
Steve Roemerman is the chairman and CEO of Lone Star Analysis.