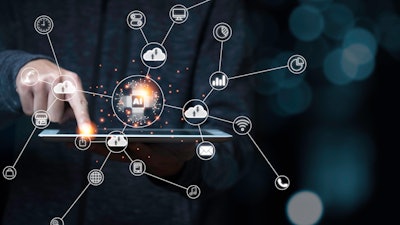
The use of Artificial Intelligence (AI) in manufacturing is growing swiftly, from an estimated market size of $2.3 billion in 2022 to an anticipated $16.3 billion by 2027 (a CAGR of 47.9 percent over the period).
Emerging from the pandemic and challenged with labor shortages, manufacturers are looking to drive efficiency wherever they can. The prevalence of industrial IoT and the search for better quality control measures have helped to drive AI adoption by manufacturers. But AI quality is a major concern. Like other types of businesses, manufacturers face challenges building high quality AI and delivering ROI on AI initiatives.
High quality ML systems have been proven to deliver real ROI in use cases such as forecasting, predictive maintenance and more. However, most manufacturers encounter significant barriers to developing high-quality ML systems, such as:
- Data science teams often lack ML development, evaluation, and testing tools that can help them build high-quality systems in a fast, agile manner, especially when faced with data challenges such as imbalanced data sets, large numbers of potential features, and variable data quality.
- Data scientists encounter challenges securing buy-in from necessary stakeholders whose approval is required to place a model into production.
- Data scientists find it hard to explain ML model behavior and errors (e.g., false positives and false negatives) to stakeholders and operators, due to the black box nature of ML systems. This inhibits trust and adoption, operational impact, and continuous mode improvement.
- Once models are in live use, ML engineering teams lack model monitoring tools to monitor and efficiently debug data quality and ML model drift issues. This makes it hard to ensure sustained performance over time.
Here are AI model quality considerations for the six most common AI use cases for manufacturers, based on our work with numerous manufacturing companies – ranging from automotive to semiconductors.
- Forecasting. Manufacturing companies have used linear models to forecast for many years, with varying success. Moving to AI models, which can be monitored and periodically tweaked to reflect changing market conditions, can help greatly improve forecast accuracy. AI model quality efforts - chiefly model testing and monitoring - can help identify and address data challenges caused by low-volume products or bad/incomplete data. They can also identify high-error segments to help data science teams iteratively improve models, and help gain stakeholder buy-in by explaining the reasons for forecast output. Finally, they help assess and debug model accuracy, drift and operational performance.
- Predictive Maintenance. While predictive maintenance is certainly not new, it’s much more accurate when driven by AI. AI model testing and monitoring can help manufacturers iteratively improve performance, address overfitting and imbalanced data set challenges. They can also help efficiently evaluate and explain quality differences across data segments. Additionally, AI quality solutions can help explain predictions to operators, to build trust, and help them take the next best action. Finally, AI model testing and monitoring can identify and explain false positives/negatives, and monitor and debug feature drift, model score drift, and the quality of data inputs. All result in more accurate predictive maintenance models - improving uptime and efficiency.
- Predictive Quality (Smart Manufacturing). When it comes to predictive quality, AI quality measures are particularly essential, helping manufacturers build high-performance models in conjunction with manufacturing process experts. They also enable much greater efficiency in evaluating multiple models trained across multiple sites, and help assess and debug overfitting and imbalanced data set challenges.
- Anomaly Detection. AI model quality efforts can help manufacturers more efficiently identify anomalies and debug conceptual soundness and performance. They can also explain why models are predicting an anomaly to improve trust in the model and achieve stakeholder buy-in.
- Warehouse Management. AI model quality efforts can aid in model selection and model performance optimization, as well as help explain predictions to operators, enabling collaboration and building trust. Finally, AI quality efforts can help monitor and debug data quality issues and ML model drift.
- Operations Automation. AI model testing in development helps assess the reliability of the model to determine the appropriate level of human supervision needed. AI model monitoring in production can provide early warning when data drift is likely to impact the accuracy of models used to automate operational processes.
Manufacturers leveraging AI who feel they have yet to see a return on their investment should explore whether AI quality efforts could move the needle – as they have in many other industries.